Large Language Models + Robots = the next “big thing”
The importance of good datasets cannot be overstated in AI.
There is groundbreaking work going on that combines natural language ability and robotics. The potential is huge, imagine having a robot that understands, thinks, plans and responds in real-time to natural language input. Or vice-versa, a ChatGPT look-a-like which learns that all that it hallucinates doesn’t correspond to physical reality and thus corrects itself, in the same way as we humans do. Among all the actors in the robotics field, some focus their work on the combination of large language models (LLMs) and robotics. Microsoft and OpenAI works on this (e.g. Vemprala et al, https://arxiv.org/pdf/2306.17582.pdf) as does Google. They are churning out a steady stream of high quality papers and accompanying labs and physical installations to be at the forefront of this next potential “big thing” in artificial intelligence.
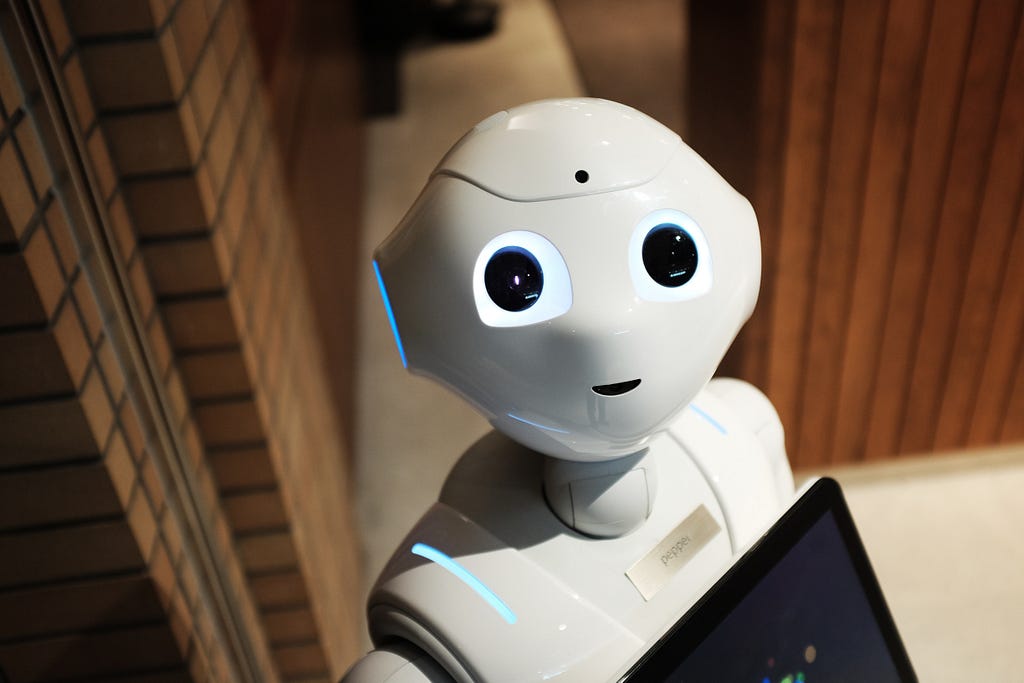
A key ingredient in previous quantum leaps in AI have been the establishment of datasets. These have been used in competitions, making results in papers reproducible, and for focusing research efforts on certain tasks. What sets Google apart, is their pursuit focused on establishing benchmarks with accompanying datasets for LLM + Robotics.
In October last year Google robotics and Stanford published dataset related work on whether a set of robot actions corresponds to a language label (Nair et al. 2022, https://arxiv.org/pdf/2109.01115.pdf ). The dataset addresses whether a set of robot actions fulfill a task, e.g. “open the left drawer”? Through crowdsourcing and video, thousands of episodes like this constitute a dataset that forms the foundation for connecting robotic action with task semantics.
In a parallel effort, during the development of the SayCan robot, Google over a year amassed close to 70000 manually assisted robot behavior demonstrations using 10 robots as well as more than a quarter of a million autonomous episodes. This extensive dataset made it possible to ground large language models to physical reality, in turn enabling robots to plan and act flexibly on commands entailing multiple steps for success (Ahn et. al, 2022 Google Robotics and Everyday Robots (https://arxiv.org/pdf/2204.01691.pdf ).
Also in October 2022, Google robotics published work on how to establish datasets for connecting language with robotic behavior. These are benchmarks for the AI and robotic community. As mentioned, high quality, public datasets tied to a task type are a critical enabler for making progress in AI. In this round, Google robotics’ dataset contains more than half a million labeled demonstrations. With this they build robots that can perform close to a hundred thousand different behaviors specified in natural language. (Lynch et al. 2022, Google robotics, https://arxiv.org/pdf/2210.06407.pdf ). Much of this work was on so-called short-horizon behavior. This prepared the ground for combining this tactical perspective with longer horizon planning.
With Palm-E in march ’23 (Driess et al. 2023, Google research and robotics, TU Berlin, https://palm-e.github.io/assets/palm-e.pdf ) we start to see the emergence of robots that combine language and sensory input as a new multimodal “language”. Some of the “words” in the sentence are sensory input. This is used as input in using and training a model. The output is text that can be interpreted and executed by a robot. This groundbreaking methodical work relies among other things, on the aforementioned dataset work of Lynch et al. The net result is a fairly long step towards machine learning models that are natural language speakers and at the same time better grounded and relating to and affecting the physical world.
Although a year old, this clip sets the context and explains fairly well where we’re headed; https://www.youtube.com/watch?v=dCPHGwW9SOk .
Computas has long experience with AI, we presently work with clients on large language models (LLMs), and we’re really good at working methodically with datasets. Talk to us if you have interesting challenges.
Large Language Models + Robots = the next “big thing” was originally published in Compendium on Medium, where people are continuing the conversation by highlighting and responding to this story.